Kserve - Inference with PVC using nfs
Environment
Kubernetes version: v1.22.7
kubeflow version: v1.6.0
kubeadm single cluster
cni: flannel https://github.com/flannel-io/flannel
k8s cluster reference: https://mlops-for-all.github.io/docs/setup-kubernetes/kubernetes-with-kubeadm/
4.3. Install Kubernetes - Kubeadm
1. Prerequisite # 쿠버네티스 클러스터를 구축하기에 앞서, 필요한 구성 요소들을 클러스터에 설치합니다. Install Prerequisite을 참고하여 Kubernetes를 설치하기 전에 필요한 요소들을 클러스터에 설치해
mlops-for-all.github.io
Train and freeze the Sklearn model
from sklearn import svm
from sklearn import datasets
import joblib
def train(X, y):
clf = svm.SVC(gamma='auto')
clf.fit(X, y)
return clf
def freeze(clf, path='./'):
joblib.dump(clf, f'{path}/model.joblib')
return True
if __name__ == '__main__':
iris = datasets.load_iris()
X, y = iris.data, iris.target
clf = train(X, y)
freeze(clf)
Persistent Volume (Network File System)
---
apiVersion: v1
kind: PersistentVolume
metadata:
name: nfs-storage
labels:
type: nfs
spec:
capacity:
storage: 10Gi
volumeMode: Filesystem
accessModes: ["ReadWriteMany"]
mountOptions: ["hard"]
nfs:
server: nfs-server-ip.or.domain
path: nfs/server/path # sklearn model.joblib saved path
위 PV 컨피그 정보를 kubectl로 적용한다.
Persistent Volume Claim
---
apiVersion: v1
kind: PersistentVolumeClaim
metadata:
name: nfs-storage-claim
spec:
storageClassName: "" # 빈 문자열
accessModes: ["ReadWriteMany"]
resources:
requests:
storage: 4Gi
selector:
matchExpressions:
- key: type
operator: In
values:
- nfs
위 PVC 컨피그 정보를 kubectl로 적용한다. namespace는 kubeflow-user-example-com로 지정한다.
apiVersion: serving.kserve.io/v1beta1
kind: InferenceService
metadata:
name: sklearn-from-pvc
spec:
predictor:
model:
modelFormat:
name: sklearn
storageUri: "pvc://nfs-storage-claim/model.joblib"
위 InferenceService 컨피그 정보를 kubectl로 적용한다. namespace는 kubeflow-user-example-com로 지정한다.
그럼, kubeflow-user-example-com namespace에 Pod가 생성되며 3개의 컨테이너가 모두 running 될때까지 기다린다.
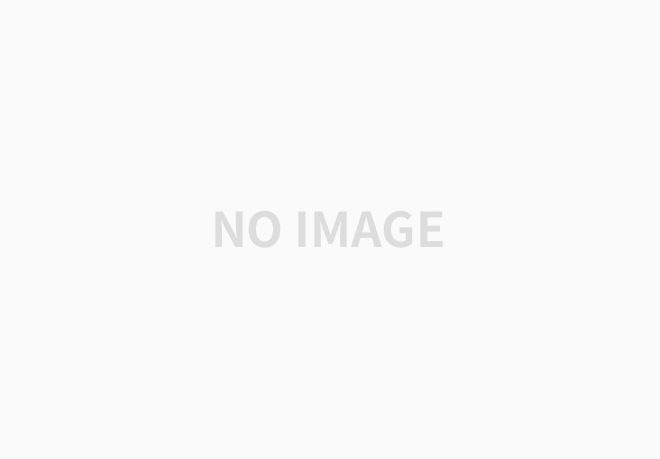
kubeflow model server UI에 위와같이 모델이 생성중으로 뜨고, Pod가 모두 running 상태가 되면, 아래 sklearn-iris 모델과 같이 체크표시가 된다.
마지막으로, istio-ingressgateway 서비스의 80:30464/TCP 포트포워딩 정보를 통해
curl --location --request POST 'http://{cluster ip}:{port}/v1/models/sklearn-from-pvc:predict' \
--header 'Host: sklearn-from-pvc.kubeflow-user-example-com.example.com' \
--header 'Cookie: authservice_session={authservice_session_token}' \
--header 'Content-Type: application/json' \
--data-raw '{
"instances": [
[6.8, 2.8, 4.8, 1.4],
[6.0, 3.4, 4.5, 1.6]
]
}'
위 curl 명령어로 올바른 header 값을 넣고 요청하게 되면 정상적으로 작동하는 것을 확인할 수 있다.
*문제점
PV생성할 때, spec.nfs.path 에 모델이 위치한 depth를 명시하지않고 더 상위 depth를 명시한 뒤,
InferenceService 생성할 때, storageUri를 {mount_path}/model/dir/path/model.joblib이라고 명시하면
Exception: Cannot locate source uri /mnt/pvc/jeawoo0594/ux9mba3c/1 for PVC 라고 에러가 뜨면서 되질않는다... 원인아시는분은 댓글 좀 부탁드립니다.ㅠㅠㅠㅠ